Introduction
In the current fast-paced, data-driven business world, companies are always trying to find ways to improve their efficiency, decrease the amount of waste, and increase the level of customer satisfaction. Predictive quality analytics provide businesses with a powerful means to reach their objectives via historical data analysis and sophisticated statistical models. With predictive quality analytics, businesses are better able to anticipate any problems before they arise resulting in improved product quality as well as operational efficiencies.
In this article we’ll go into the notion that predictive quality analytics can be used to identify issues. We’ll discuss how it operates and why it is becoming a vital element of business strategies in the modern era. Additionally, we’ll look into real-world examples of case studies that demonstrate the impact that the use of predictive quality analytics has had in various fields.
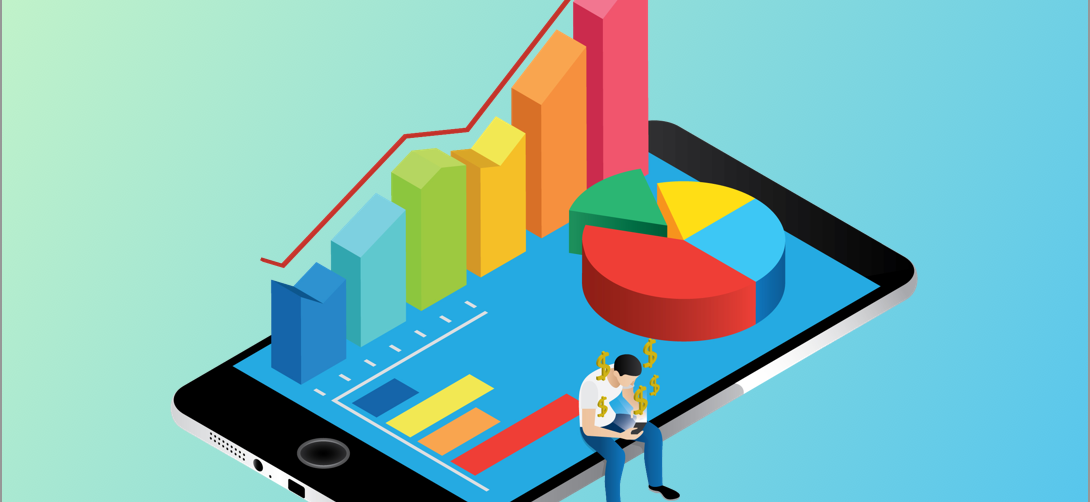
The Growing Importance of Predictive Quality Analytics
Predictive quality analytics is focused on the use of historical data, such as machine learning, data, and statistical models to forecast future outcomes. In contrast to traditional techniques of quality control that react when they arise, the predictive approach takes the proactive route. Through the analysis of patterns in previous information, it is able to identify the possibility of issues that may cause problems with product quality or operational efficiency. The forward-thinking approach allows companies to take informed decision-making and corrective actions prior to issues becoming significant.
Predictive quality analytics help businesses in many ways. This includes making products more reliable, reducing the number of defects, cutting costs, and increasing satisfaction with customers. For instance, when manufacturing businesses can employ predictive analytics to analyze production lines continuously and determine the likelihood of equipment being damaged or if an entire batch of goods could not meet the standards for quality. This kind of warning system will prevent expensive interruptions and help ensure customers get high-quality goods.
How Predictive Quality Analytics Works
Predictive quality analysis involves gathering and analyzing large amount of data collected from a variety of sources, such as sensor readings, production logs as well as maintenance records, and customer feedback. Modern algorithms analyze this data in order to discover patterns and patterns that could suggest quality problems. The main ingredient of predictive analytics is the use of machine learning. In this process, the system continuously acquires fresh data and improves its prediction over time.
When the system is able to identify an issue that could be causing concern and has notified the concerned personnel, like the quality controllers or production managers teams, this allows them to address the problem prior to the situation escalating. It could involve changing procedures, replacing defective equipment as well as addressing the lack of training between employees. It is the ultimate aim to eliminate defects, decrease loss, and ensure items meet standard of quality.
The Benefits of Predictive Quality Analytics
There are many strong reasons that predictive quality analytics are increasing in demand across various areas like manufacturing, healthcare and retail. We’ll give an overview of some most important benefits:
Cost reduction: By identifying and fixing problems with quality before they arise, firms can dramatically reduce the costs of return, defects, and the need to rework. It results in increased profitability as well as improved efficiency in operations.
Enhance Product Quality: by The use of predictive quality analytics can help companies keep their product quality consistent by detecting potential problems at an early stage of the production process. The result is fewer errors as well as better satisfaction for customers and a stronger loyalty to the brand.
Reducing downtime: in businesses that are dependent on machines and equipment such as predictive analytics, predictive analytics are able to predict what time a machine is most likely to malfunction, allowing companies to plan maintenance. This can reduce the chance of unexpected downtime and make sure that operations run smoothly.
Utilizing Predictive Analytics: Organizations using predictive analytics are able to access valuable information which aids decision-making processes. Management now makes data-driven decisions rather than basing their choices off intuition or outdated data sources.
Optimized Resources: By predicting the time when resources are required, companies can improve the supply chain of their business, decrease wasted resources, and improve the management of inventory levels. This will ensure that the correct products and services are on hand at the time needed, with no running out of stock or shortages.
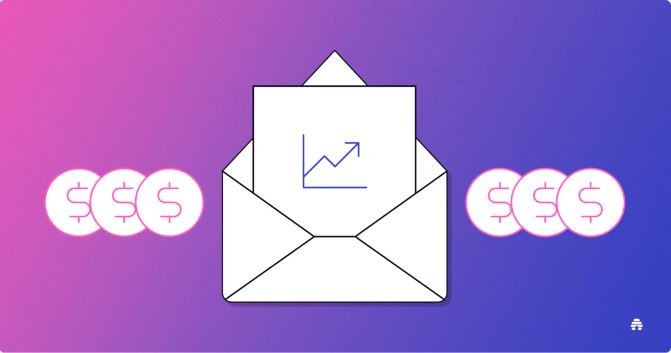
Real-World Case Studies
We’ll now look at some real-world cases to see the way predictive quality analytics are helping to improve the quality of life in different areas.
Manufacturing Sector:
A major auto manufacturer has employed predictive quality analysis to ensure the health of their manufacturing line. Through the use of sensors and real-time data analytics, the business could predict which machines might be unable to function. Planned maintenance helped them significantly decrease time to repair by 30 percent and identify quality issues early. Once detected, corrective action were taken before customers noticed issues affecting product quality.
Healthcare Industry:
In the field of healthcare the use of predictive quality analytics is utilized to enhance the quality of care for patients and cut costs. The largest hospital network employed predictive analytics to study patient information and forecast the possibility of complications following surgery. Doctors were able to use proactive steps taken by physicians in this hospital in order to enhance patient results, reduce readmission rates and save millions in healthcare costs while improving its image as an exceptional provider of high-quality health services.
Retail Sector:
An important retailer has utilized predictive quality analysis to improve its management of inventory and minimize product quality defects. Analyzing sales history and feedback from customers they were capable of predicting which items might be susceptible to defects in quality. It helped to address possible problems before they impacted customers and decreased the amount of returned products. In the end, customers were more satisfied and reduced the cost that came with returning.
My Experience with Predictive Quality Analytics
I’ve witnessed the transformational effect of predictive quality analytics on my company’s operations. As soon as we introduced predictive analytics into our manufacturing process, I was taken aback at how drastically downtime decreased. Machine breakdowns used to cause production stoppage for hours; now through predictive analytics it was possible to predict when one might need servicing instead of incurring expensive interruptions and costly interruptions.
My biggest takeaway from predictive analytics was seeing its benefits go beyond simply saving money: by anticipating problems before they became major issues or potential areas for improvement early enough, we were able to make better products with reduced defects that increased customer satisfaction and loyalty resulting in greater satisfaction from both existing customers as well as future ones.
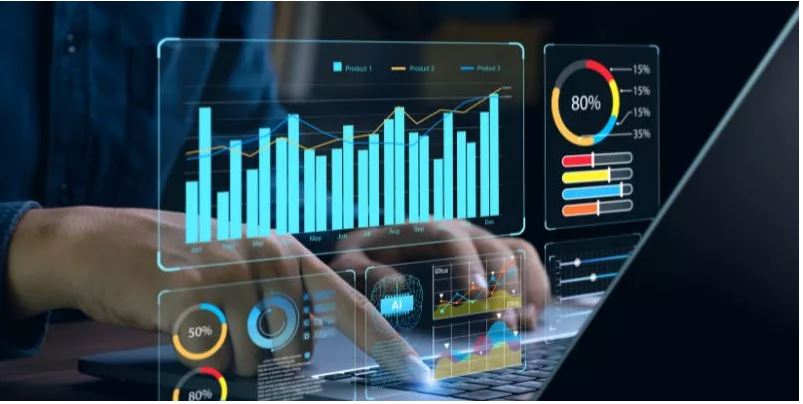
Challenges of Implementing Predictive Quality Analytics
Predictive quality analytics offer advantages to organizations; however, organizations must overcome some challenges in using it effectively. One major barrier lies with accessing high-quality information; predictive analytics relies on current and accurate information in order to offer forecasts which provide solid predictions; however if insufficient or outdated or inaccurate data is used it could result in inaccurate forecasting leading to costly errors.
A lot of companies already have processes, systems, and software in place, So the process of integrating predictive analytics into existing workflows is time-consuming and expensive. This could require adjustments for IT infrastructure, training for staff as well as a culture change within the organization.
My Thoughts on Overcoming These Challenges
In my personal experiences, I’m convinced that the solution to these issues is possible when you take the proper strategy. The first step is to be sure that the data taken is accurate, clean, and reliable. Investment in data management systems and instruments can be an essential step toward improving data accuracy as well as guaranteeing predictions are made using reliable information.
Engaging all departments in the implementation process is also key for surmounting integration hurdles. It involves working in close collaboration with IT teams as well as quality control groups and others to make sure everyone is on the same page and is aware of the importance of predictive analytics. Once everyone is involved, it becomes easier.
Benefits of Predictive Quality Analytics:
This article presents various advantages prescriptive quality analysis brings to companies. Predictive analytics assists organizations by increasing product quality while simultaneously decreasing delays and defects as well as optimizing resource use efficiencies. People are curious as to the possibilities the technology holds for improving overall bottom lines for businesses and increasing revenues.
Real-World Applications:
Our readers want to know actual examples and case studies that demonstrate how the use of predictive data can be applied to different sectors. This article showcases examples of companies from retail, manufacturing and healthcare as well as other industries who have successfully adopted predictive analytics into their workflows. This piece also details issues they encountered along the way and tangible outcomes like improved efficiency, reduced resources loss or customer satisfaction that resulted.
Challenges and Solutions:
Implementing predictive quality analyses can be challenging for businesses. This article highlights common challenges encountered when trying to implement such analyses, including data quality issues as well as resistance from cultural backgrounds. Practical strategies and solutions are provided for overcoming such hurdles with tips on how to reap their benefits by adopting predictive analytics technologies successfully.
Future Trends:
As technology rapidly develops, people have grown more intrigued with learning more about predictive analytics for quality. The article discusses the latest developments in this field, including the increased use of artificial intelligence (AI), learning (ML), and prescriptive maintenance. The topic examines how advances in these areas change the standards of industry as well as advancing predictive capabilities opening new avenues for companies to invent and remain competitive.
Personal Insights:
The topic offers an intimate view by giving insights and experience from professionals in the field who have direct experience in the use of predictive quality analytics. This book provides practical guidance as well as lessons learned strategies for successful implementation that are that are based on real-life observations. People are keen to know about the experiences of these experts and learn about their struggles as well as their successes and the effect predictive analytics have had on their companies.
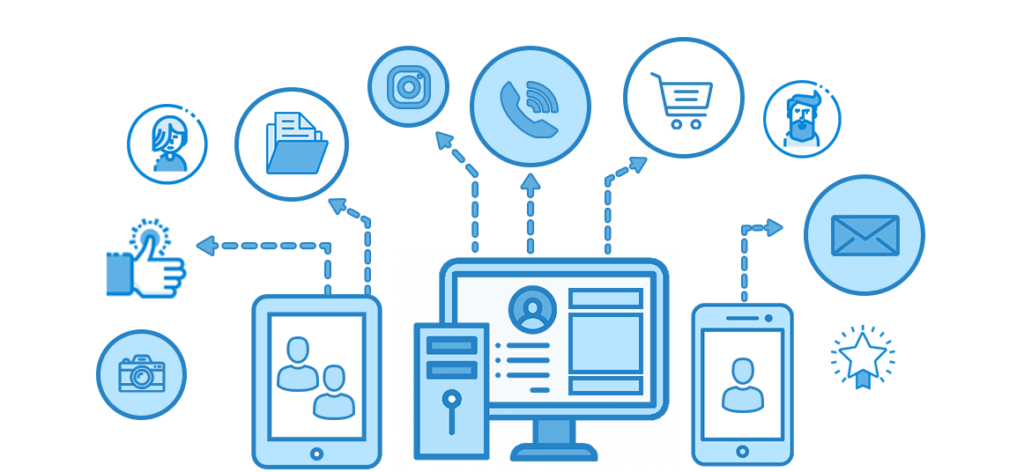
Conclusion
Predictive quality analytics is an integral component for businesses aiming to outdistance competitors with superior goods and services. Anticipating potential issues allows organizations to reduce costs, enhance product quality, make informed decisions more quickly, and be ahead of competitors by anticipating them prior to happening. Although there are some challenges associated with applying predictive analytics, the advantages more than outweigh any drawbacks particularly when the proper system and data are set up.
When more companies adopt predictive, high-quality analytics, the technology will continue to develop and deliver even better capabilities. Firms that take advantage of this technology today will be well-placed to prosper in an ever highly competitive and information-driven environment. If you’re still not employing predictive analytics in your organization, now’s an ideal time to begin exploring the potential of this technology.
FAQS
What exactly is predictive quality analytics?
This research question aims to clarify predictive quality analytics. Then, it explains the purpose behind it and how it differs from standard quality control strategies.
What can predictive quality analytics do to enhance the quality of products?
The readers want to know the ways that predictive analytics helps improve the quality of products and services offered by businesses.
What sectors can most benefit from predictive quality analytics?
This guide reveals which industries that have experienced the biggest benefit from the implementation of predictive quality analysis and how those industries benefit the most.
What are the most important factors in predictive quality analysis?
It covers the most important components and the technologies that are used in predictive analytics like data collection and machine learning algorithms and modeling predictively.
What can predictive quality analytics do to help reduce cost?
The readers are keen to know how predictive analytics help companies reduce costs associated with problems, downtime as well as maintenance and operating inefficiencies.
What are the difficulties of the implementation of the predictive quality analysis?
This article addresses the common challenges companies face when implementing predictive analytics. This includes problems with data integration, culture resistance, as well as the necessity to hire skilled workers.
Do you have any instances of predictive quality analytics working?
The reader will find practical examples and case studies that show how businesses are able to implement predictive analytics that has led to tangible gains in productivity and quality.
What can companies do to get started with an analytics system that can predict quality?
This FAQ offers practical tips and suggestions for companies looking to get started with predictive analytics. This includes making plans, preparing data as well as selecting appropriate software.
What will the trends of the future be in the field of predictive quality analytics?
It examines emerging advancements and emerging trends within the field, like the incorporation into AI, IoT (Internet of Things) and predictive maintenance and the potential implications for the business strategy.
What ethical implications are there in using predictive quality analysis?
This issue addresses ethical concerns regarding the privacy of data, biases in algorithms, and the ethical use of predictive analytics for decision-making.