Introduction
Supply chains face constant pressure in today’s globalized markets to be more efficient, faster and cheaper. Predictive analytics supply chain case study optimization has become a priority for businesses, thanks to the use of predictive analytics. This technology allows them to improve their decision-making and anticipate potential disruptions. This case study highlights how predictive analytics has been implemented into a real supply chain. It also highlights challenges, strategies and results.
Supply chain management shifts from reactive to proactive, changing operations in procurement, manufacturing and inventory management. We’ll dive in to the specifics of how one business used predictive analytics for its supply-chain performance.
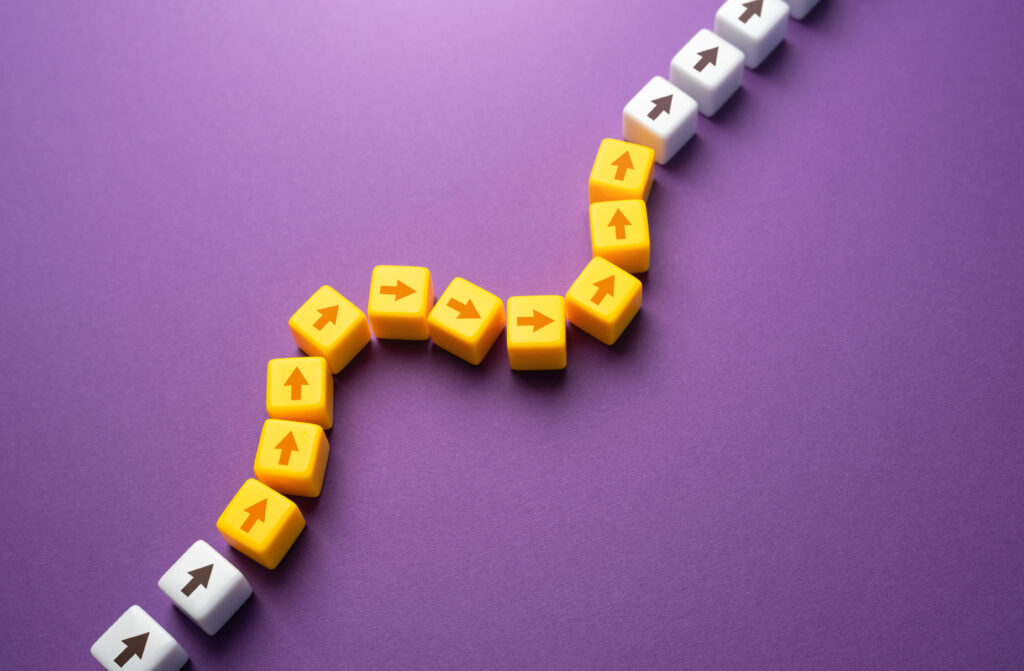
Growing costs and operational inefficiencies are the problem
A mid-sized producer of electronic goods faced increasing costs, delays and the inability to accurately forecast demand from customers.Due to an unstable market, traditional inventory and demand management techniques were unsuccessful; some of the main issues were:
- Unexpected delivery delays have a negative influence on both by causing missed deadlines and eroded customer confidence.
- Supplier Risk: Your company’s operations are at risk when there are disruptions from important suppliers since they are largely responsible for manufacturing delays and disruptions.
- Demand volatility – Predictions of sales can fluctuate by up to 20%. It leads to shortages and excess inventory.
In order to address these problems, the company chose to use predictive analytics. Its goal was to identify patterns and risks in order to streamline operations, reduce risk, and mitigate them.
It’s time to implement predictive analytics
A data analytics company was partnered up with the firm in order to develop a solution for predictive analytics that met its specific needs. Implementation was divided into three phases.
- Data collection: Historical data from CRM systems, ERP platforms and IoT devices. Included were demand patterns, production plans, and shipping records.
- Model development: To predict fluctuations in demand, possible supplier delays and optimal stock levels, machine learning models have been trained.
- Integration: The company integrated predictive insights into its existing supply-chain management software to enable real-time decisions.
The key tools were Tableau and Python for visualizing data, as well as APIs to allow seamless integration. Workshops were also held to teach employees how to interpret predictive insights, and adjust operations accordingly.
Measureable outcomes: Real-World impact
The company saw significant improvement in the performance of its supply chain within six months after implementation:
- Inventory Optimization: Inventory costs were cut by 15% through improved forecasting and reduced safety stocks, and delivery times improved 20 percent as a result of predictive modeling that reduced logistical disruptions while simultaneously improving on-time deliveries by 20%.
- Supplier Risk Mitigation : Early identification of possible supplier delays led to proactive measures that reduced downtime by 30%, not only improving operational efficiency but also customer satisfaction and profit potential.
The results of these improvements not only improved operational efficiency, but also customer satisfaction and profitability.
What I think about Predictive Analysis
When I heard the term predictive analytics for the first time, I was sceptical about its potential to impact supply chain operations. As I dug deeper into the topic, I began to see how data and technology could be combined in a way that would allow me to anticipate issues ahead of time.
Predictive models are a fascinating way to turn raw data in real-world insights. The ability to avoid problems, rather than just respond to them, was an important game changer for me.
I have always been a believer in leveraging technology to grow a business. I was impressed by the way predictive analytics transformed this business. This reinforced my conviction in data-driven decisions.
Predictive analytics empowers the people, not just operations. When employees have clear insight into their decisions, they feel more confident.
Predictive analytics would be a key component of my strategy if I was leading a supply-chain today. This is no longer an option, but rather a requirement to stay competitive.
Reflecting on this case, I am reminded that every problem in the supply chain is an opportunity to innovate. This mindset is epitomized by predictive analytics.
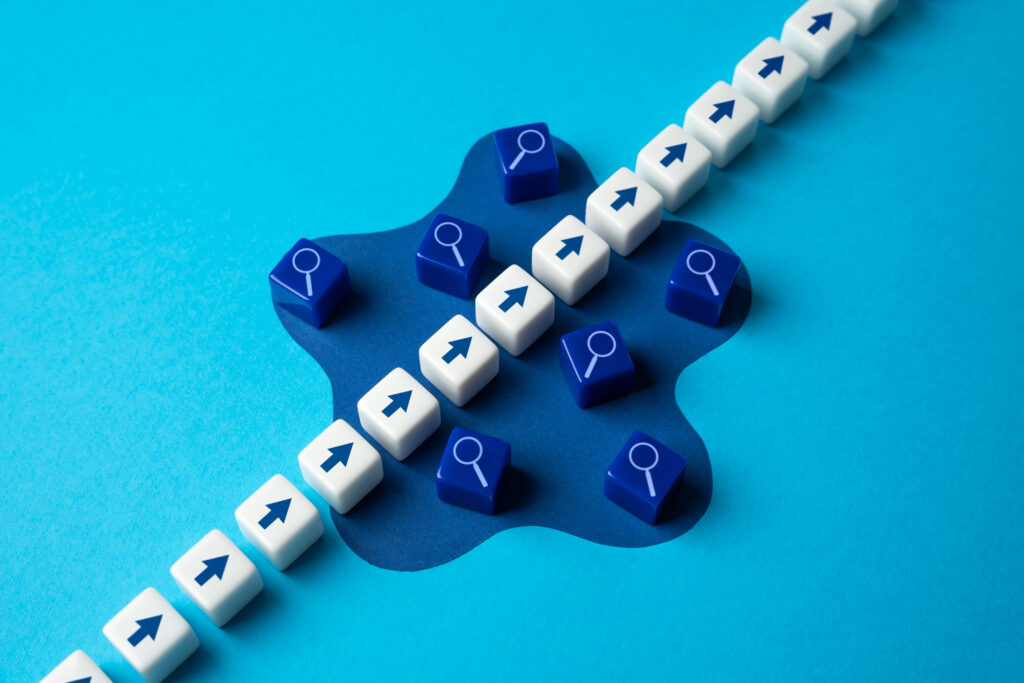
Applications across industries
The use of predictive analytics is not limited to the electronics sector.Numerous industries are impacted.Predictive modeling, for instance, lowers markdowns for seasonal products in retail.
- Healthcare Analytics: Increase inventory to guarantee availability and cut down on waste.
- Automotive: Identifying equipment faults and saving money are possible with predictive maintenance.
These illustrations show how supply chain problems can be resolved with predictive analytics.
Learnings and best practices
Implementing predictive analytics requires planning and execution. This case study contains:
- Clean, accurate data and relevant information are crucial for the development of effective models.
- Collaboration between IT and Operations: You can ensure smooth integration by aligning your IT team with the operations and management teams.
- To remain effective, predictive models need to be updated regularly with new data.
- Employees need to be supported and trained in order to adopt data-driven processes.
Other companies can benefit from these practices to maximize their predictive analytics value in supply chains.
Resilience of supply chains:
Supply chain resilience is basically the capability of a system to prepare, anticipate, react to and recover from interruptions while minimizing impact to business performance. Diversifying your suppliers, investing into robust logistics, and using predictive analytics are all ways to prepare for potential disruptions. It is very important to create a resilient supply chain, which can handle shocks such as economic changes, geopolitical tensions or natural disasters.
Sustainable Initiatives
Sustainable supply chains entail efforts to limit environmental impact through waste reduction, resource optimization and greenhouse gas emission reduction efforts, ethical practice enforcement across supply chains as well as ethical supplier selection practices.
Digital Transformation and Predictive Analysis:
The digital transformation of supply chain is the use of technologies such as IoT, AI, and predictive analytics to increase the effectiveness and efficiency of the operations. Predictive analytics employs ML and statistical algorithms to predict future events with high probabilities of success. It can be used to help businesses forecast demand accurately, improve inventory levels and supply chain planning. This will lead to lower costs and greater responsiveness.
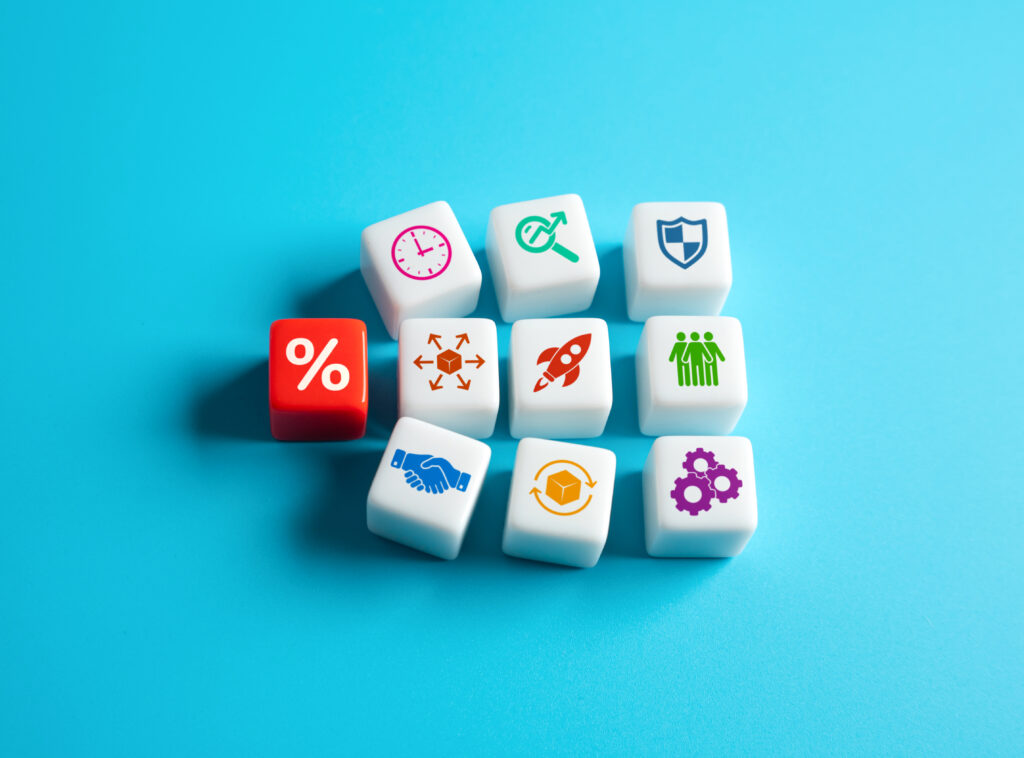
AI and Machine Learning:
AI and ML is also applied in inventory management and maintenance of logistics infrastructure. The AI improves operational efficiency and decision-making, which allows companies to ship products faster and more reliably.
Analytics Platforms Investment:
Investors are investing more in platforms for advanced analytics that combine data from different points along the supply chain. They allow for real-time analysis of data, which can then be used quickly to inform decisions. Big data analysis helps companies identify market trends and predict changes. It also allows them to respond more quickly to customers’ demands.
Flexible Supply Chains:
Flexible supply chain management is the ability to quickly adapt to changing market conditions or operational environments without significant performance losses. It is important to be able to adjust operations to meet changes in demand and supply. This may include switching suppliers or changing production methods. In industries with volatile market conditions or short product cycles, flexible supply chains can be especially important.
Digital Twins
The digital twin is a major advancement in the management and optimization of supply chains. Digital twins are virtual models of processes, products, and services. The pairing of virtual and real worlds allows for system monitoring to prevent problems from occurring, create new opportunities and plan using simulations. The digital twins enable companies to create highly accurate simulations for supply chain logistics and production processes. They can also simulate distribution networks. Models are created to simulate and predict outcomes, and optimize dynamically operations.
Blockchain Technology
Further exploration is taking place into how blockchain technology could use transparency to foster trust within supply chains. Blockchain provides a secure and transparent record to consumers by providing a ledger decentralized that records transactions in a network.
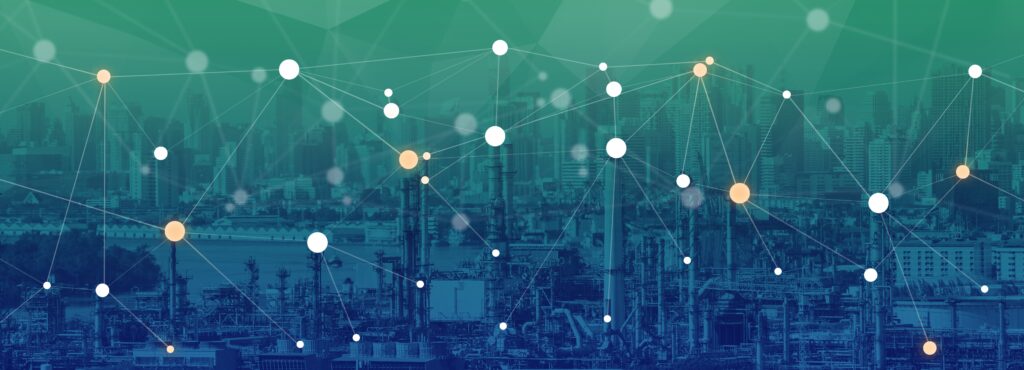
Conclusion
The use of predictive analytics has revolutionized supply chain management. It allows businesses to better anticipate and manage challenges. This case study shows the benefits of using predictive analytics: reduced costs, increased efficiency and improved customer satisfaction. Adopting predictive analytics to stay competitive is essential as global markets are becoming more unpredictable.
I have reflected on my experience and am now convinced that predictive supply chain analytics are the future. It is an extremely powerful tool that not only solves problems but promotes growth and innovation. This transformational approach offers endless opportunities to companies who are willing.
FAQs
1.What is the predictive analytics of supply chain management?
Predictive Analytics is the use of historical data, statistical models and other tools to predict future conditions in supply chains and help businesses make better decisions and optimize their operations.
2. What is predictive analytics and how does it improve resilience in the supply chain?
Predictive analytics improves recovery from interruptions in the supply chain by allowing businesses to forecast potential disruptions, and develop proactive strategies for mitigating risks.
3.How does AI affect logistics and supply-chain management?
AI improves efficiency in supply chains by automating and optimizing processes like inventory management, route-planning, and predictive maintenance.
4.How can digital twins help supply chains?
Digital twins can simulate the supply chain assets and processes to plan and predict better strategies and improve operational efficiency.
5.What are the benefits of predictive analytics for companies?
Companies can improve service, decrease wait times and better tailor their inventory by improving demand prediction and delivery accuracy.
6. What are some of the challenges associated with implementing supply chain predictive analytics?
The challenges include managing privacy issues, updating models continuously, and interpreting analytics results.